Situation
A premium automobile manufacturer in the U.S. faced inefficiencies in its core inventory management system. Existing practices led to excess inventory levels, creating the need for an additional storage unit. This situation required a scalable solution that could optimize core inventory while ensuring demand-based forecasting.
Challenge
The client needed to address:
- Inefficient utilization of existing storage facilities.
- Maximizing returns on core part storage.
- Accurately predicting core demand to avoid unnecessary expansion costs.
Approach
Mu Sigma employed its proprietary problem-solving frameworks, muUniverse and muPDNA, to map the interconnected problem space and identify inefficiencies within the supply chain. Collaborating with the client’s core engineering team, we deep-dived into their demand-supply statistics to address gaps at the core level:
scalable demand-driven inventory management framework:
Identifying Gaps in Demand Data: Our initial demand analysis revealed that the client had demand-supply statistics at a Part level (e.g., engine, transmission) but lacked visibility at a Core level (e.g., pistons, cams, connecting rods).
Mapping Parts to Cores: To address this, we collaborated with the client’s core engineering team, including design release engineers, and obtained comprehensive bills of materials for all parts. Using this information, we converted part-level demand into core-level demand by calculating the part-to-core ratio.
Forecasting Demand Across Model Years: Core demand was segregated under different model year buckets, enabling more precise demand forecasting at the category and model year levels.
Implementing Hybrid Forecasting Models:
A Regression-based approach was used for near-term predictions by leveraging vehicles in operation (VIO) data, delivering accurate forecasts for short-term inventory needs.
For long-term predictions (spanning five years), we employed an ARIMAX model, combining linear regression and time-series analysis to account for both seasonality and VIO volume changes.
Defining Inventory Banks: With demand forecasts in place, we quantified core inventory requirements and determined minimum and maximum inventory levels for the next five years. Key considerations included lead times for cores entering the system and fluctuations in demand.
The result was a robust, scalable solution that aligned inventory management with business goals, optimizing storage and driving efficiency across the supply chain
Impact
Our analytics-led approach helped them identify excess core inventory to the tune of several million dollars and streamlined the inventory management process to ensure optimal use of storage facilities.
The outcomes we enabled include:
- Avoided the need to lease an additional storage facility, saving up to $500,000 annually
- Terminated the lease of an existing storage facility, adding another $500,000 in yearly savings.
- Disposed excess inventory of 17.6K core inventory resulting in cash inflow of $5.6M
Business Impact
-
$500,000
Saved on Storage
-
176 M
Worth Excess Inventory Disposed
Let’s move from data to decisions together. Talk to us.
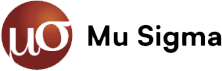
The firm's name is derived from the statistical terms "Mu" and "Sigma," which symbolize a
probability distribution's mean and standard deviation, respectively.