Situation
A leading computer manufacturer recently outsourced its factory operations. But with most processes being manual, there were significant variations in production across multiple product lines, resulting in excessive backlogs and long cycle times.
A glaring communication gap between the Sales and Operations teams stemming from a lack of clear understanding of the plant’s actual production capacity. Product line allocations were being made on an ad hoc basis leading to inefficient production schedules and a growing backlog of orders.
Objectives
The client sought a data-driven approach to:
- Reduce backlog and shorten production cycle time across product lines.
- Reduce workforce overtime, which had become the norm.
Solution
We implemented simulation modeling to improve factory planning and decision-making. The solution was developed in a phased manner, ensuring accurate data collection, validation, modeling, and continuous learning.
Step 1: Data Mapping
- Identified factories across the globe with similar processes to map data.
- Conducted time-study analysis , deriving average cycle times week over week across processes.
- Mapped process data from identified factories that were aligned with the client’s factory operations.
- Using mapped data, the factory was modeled and refined through multiple iterations based on evolving process understanding.
Step 2: Identifying & Implementing Key Levers
- Defined key operational levers to observe systemic behaviors and identify inefficiencies.
- Integrated lever functionality into the simulation model to predict process changes dynamically.
- Validated the model’s behavior against actual factory operations to ensure accuracy.
Step 3: Understanding Process Details & Distribution Fitting
- Engaged stakeholders and experts to capture granular process insights.
- Made informed assumptions where data was unavailable.
- Fitted over 360 unique distributions to represent 20 product lines across 15 different processes.
Impact
- 85% model accuracy: The simulation was validated with high precision against actual factory operations.
- 27% potential annual reduction in backlog after adopting the model. Analysis revealed smaller, previously unnoticed inventory build-ups that contributed to delays.
- The Supply Chain Operations team invested in scaling simulation modeling across multiple factories and hubs in North America.
Business Impact
-
85%
Validated model accuracy
-
27%
Reduction in potential annual backlog
Let’s move from data to decisions together. Talk to us.
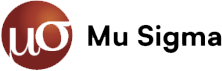
The firm's name is derived from the statistical terms "Mu" and "Sigma," which symbolize a
probability distribution's mean and standard deviation, respectively.